Artificial Intelligence (AI) and Machine Learning (ML) are two interrelated concepts that involve the development of computer systems capable of performing tasks that typically require human intelligence. While AI is a broader field encompassing various techniques and approaches, ML is a subset of AI that focuses on algorithms and models that enable computers to learn and improve from data without explicit programming.
Artificial Intelligence involves the development of intelligent systems that can perceive their environment, reason, learn from experience, and make decisions or take actions to achieve specific goals. AI systems can exhibit characteristics such as natural language processing, problem-solving, knowledge representation, and machine perception.
Machine Learning, on the other hand, is a specific approach within AI that emphasizes the ability of machines to learn from data without being explicitly programmed. ML algorithms enable computers to automatically analyze large datasets, recognize patterns, and make predictions or decisions based on the identified patterns. ML algorithms can improve their performance over time through experience and exposure to new data.
In essence, AI aims to create intelligent systems that can mimic human intelligence, while ML focuses on developing algorithms and models that allow machines to learn and make predictions from data. ML is a key component of AI, as it provides the means to train AI systems and enable them to adapt and improve their performance based on the available data. Together, AI and ML have the potential to revolutionize various industries and solve complex problems by leveraging the power of data and intelligent algorithms.
Applications of Artificial Intelligence (AI) and Machine Learning in different Industries
Artificial Intelligence (AI) and Machine Learning (ML) have found diverse applications across various industries, revolutionizing the way businesses operate and transforming traditional practices. From healthcare and finance to manufacturing and retail, AI and ML have paved the way for improved efficiency, enhanced decision-making, and the development of innovative solutions.
AI and ML are being extensively employed in the healthcare sector for a wide range of applications. In supervised learning, the algorithm undergoes training using labelled data, where each data point is linked to a corresponding label or target value. Medical professionals can leverage AI algorithms and ML models to analyze patient data and detect patterns that may assist in early disease diagnosis. Additionally, AI-powered systems can aid in medical imaging analysis, enabling accurate and efficient interpretation of scans and identifying anomalies that might otherwise be missed.
The finance sector has also witnessed significant advancements with the implementation of AI and ML technologies. AI algorithms are employed for tasks such as fraud detection, risk assessment, and algorithmic trading. ML models analyze vast amounts of financial data to predict market trends, optimize investment strategies, and improve portfolio management. Furthermore, AI-powered chatbots and virtual assistants are utilized to provide personalized customer support, streamline banking operations, and enhance the overall user experience.
Manufacturing processes have been greatly transformed by AI and ML. AI-powered systems monitor and analyze real-time data from sensors, enabling predictive maintenance and minimizing equipment downtime. ML models optimize production lines, improving efficiency and reducing costs. Robotics and automation, driven by AI, have revolutionized assembly lines and logistical operations, enhancing productivity and accuracy. AI and ML also play a crucial role in quality control, identifying defects and anomalies in products.
The retail industry has embraced AI and ML to enhance customer experiences and optimize operations. AI algorithms analyze customer data, including browsing and purchase history, to provide personalized product recommendations. This improves customer satisfaction and drives sales. ML models are also used for demand forecasting and inventory management, ensuring optimal stock levels and reducing inventory costs. Additionally, AI-powered chatbots are employed to provide instant customer support, address queries, and facilitate online transactions.
The transportation and logistics sector has greatly benefited from the implementation of AI and ML technologies. AI-powered algorithms optimize routing and scheduling, reducing fuel consumption and improving delivery efficiency. ML models analyse historical data to predict demand patterns, enabling better resource allocation and reducing transportation costs. Furthermore, AI is driving the development of autonomous vehicles, revolutionizing transportation by improving safety, efficiency, and accessibility.
In the field of cybersecurity, AI and ML are crucial tools in detecting and preventing cyber threats. AI algorithms can analyze network traffic and identify suspicious patterns or anomalies, helping to detect and respond to potential cyber attacks in real-time. ML models learn from historical data to continuously improve threat detection and develop more effective security measures.
Overall, the applications of AI and ML in various industries are extensive and continue to expand. From healthcare and finance to manufacturing, retail, transportation, and cybersecurity, these technologies have the potential to optimize processes, improve decision-making, and drive innovation. As advancements in AI and ML continue, businesses across industries will undoubtedly harness their power to stay competitive and meet the evolving needs of the modern world.
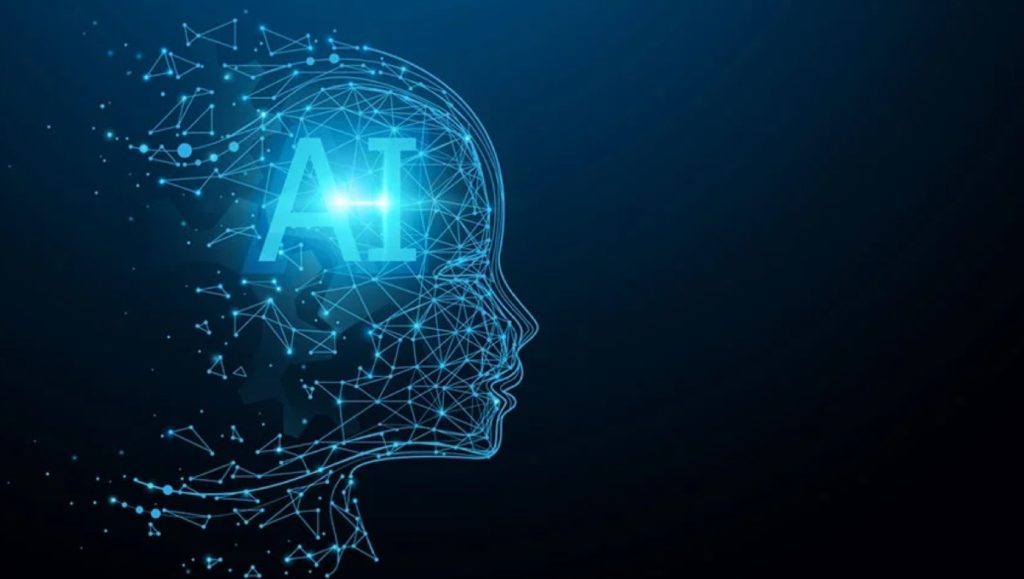
Advantages and Benefits of AI and ML
Artificial Intelligence (AI) and Machine Learning (ML) offer numerous advantages and benefits across various industries. One key advantage is the automation of repetitive tasks, leading to increased efficiency and productivity. AI and ML technologies can handle mundane and routine activities, freeing up human resources to focus on more complex and value-added tasks. This not only improves operational efficiency but also enables employees to dedicate their time and expertise to more critical aspects of the business.
Another advantage lies in the ability of AI and ML to analyse large volumes of data and extract valuable insights. By leveraging advanced algorithms, businesses can uncover patterns and trends that may not be apparent to humans. This data analysis capability empowers organizations to make data-driven decisions, optimize processes, and gain a competitive edge in the market. Additionally, AI and ML enable personalized customer experiences by analysing individual preferences and behaviours. This allows businesses to deliver tailored recommendations, products, and services, thereby enhancing customer satisfaction and fostering long-term loyalty.
Moreover, AI and ML offer the potential to improve accuracy and reduce errors. Machine learning algorithms continuously learn from data, enabling them to improve their performance and make more accurate predictions over time. This increased accuracy not only speeds up decision-making but also reduces the risk of human errors. Furthermore, AI and ML provide scalability and cost-efficiency. Once developed, AI systems and ML models can be easily replicated and deployed across multiple processes or systems, leading to cost savings and improved resource allocation. Lastly, these technologies drive innovation by harnessing the power of data analysis and adaptive learning, opening new possibilities for advancements and novel solutions in various industries.
Challenges AND Limitation of AI ML
AI and ML technologies, while powerful, also face several challenges and limitations that need to be addressed. Here are some key considerations:
One major challenge is related to data limitations. AI and ML models rely on vast amounts of high-quality data for effective training and accurate predictions. However, acquiring such data can be difficult, especially in domains where data is scarce or protected due to privacy concerns. Biased or incomplete data can also impact the performance and fairness of AI systems, emphasizing the need for diverse and representative datasets.
Another significant limitation is the lack of interpretability. Some AI and ML models, particularly deep neural networks, operate as complex “black boxes,” making it challenging to understand the factors influencing their decisions. This lack of transparency raises concerns about trust, accountability, and the ability to explain the reasoning behind specific predictions or actions.
Ethical considerations and potential biases are also crucial challenges. AI and ML systems can unintentionally perpetuate biases present in the training data, leading to discriminatory outcomes. Addressing bias and ensuring fairness in decision-making processes are essential to build trustworthy and inclusive AI systems.
Robustness and security are additional concerns. AI and ML models can be vulnerable to adversarial attacks, where malicious actors manipulate or deceive the system to produce incorrect results. Ensuring the robustness of models and implementing safeguards against attacks is vital to maintain the integrity and reliability of AI and ML systems.
Moreover, the computational requirements of AI and ML algorithms can be demanding, limiting their scalability and accessibility. Training complex models often requires substantial computational resources, hindering their deployment on low-powered devices or in resource-constrained environments. Overcoming these computational limitations is crucial for widespread adoption and integration of AI and ML technologies.
Finally, there is a need for ongoing research and collaboration to address these challenges and ensure the responsible development and deployment of AI and ML systems. Ethical frameworks, regulations, and standards are being developed to guide the use of these technologies, promoting transparency, fairness, and accountability.
Addressing these challenges and limitations will enable us to unlock the full potential of AI and ML, fostering their positive impact on various domains while minimizing potential risks and ensuring ethical and responsible use.
Ethical Concerns of AI ML
The rapid development and deployment of Artificial Intelligence (AI) and Machine Learning (ML) technologies have raised important ethical concerns that require careful consideration. Here are some key ethical considerations related to AI and ML:
One major concern is the potential for biases and unfairness. AI and ML systems can inherit biases from the data they are trained on, leading to discriminatory outcomes. It is essential to ensure that these technologies are developed and deployed in a way that promotes fairness, prevents discrimination, and avoids perpetuating societal biases.
Privacy and data protection are also significant ethical concerns. Safeguarding individuals’ privacy, securing sensitive information, and ensuring compliance with data protection regulations are essential to maintain trust and protect individuals’ rights.
Transparency and explain ability are critical ethical considerations Numerous AI and ML models function as intricate “black boxes,” posing difficulties in comprehending their decision-making mechanisms. There is a need for transparency in AI systems to enable users and stakeholders to comprehend how decisions are made and to address concerns related to bias, accountability, and trust.
Ethical considerations also extend to the potential impact on employment and societal well-being. AI and ML technologies have the potential to automate tasks, leading to job displacement. It is crucial to consider the social and economic consequences of AI adoption and to develop strategies for reskilling and providing new opportunities for individuals affected by these changes.
Additionally, AI and ML should be used responsibly to avoid harmful or malicious applications. Clear guidelines and regulations are needed to prevent misuse, protect against malicious use, and ensure that AI and ML systems are aligned with ethical norms and values.
Finally, the ethical development and deployment of AI and ML require multidisciplinary collaboration, involving researchers, policymakers, ethicists, and the wider society. Open dialogue, transparency, and stakeholder involvement are vital to ensure that these technologies are developed and used in a manner that benefits society while minimizing potential risks and harms.
Data privacy and security of AI
Data privacy and security are crucial considerations in the field of Artificial Intelligence (AI) to ensure responsible and ethical use of AI systems. Here are some key aspects related to data privacy and security in AI:
Protecting Personal Data: AI systems often rely on vast amounts of personal data to train models and make accurate predictions. Safeguarding individuals’ privacy rights and complying with data protection regulations are paramount. Organizations must handle personal data securely, ensuring its confidentiality and integrity, and obtain informed consent when collecting and using personal information.
Data Anonymization and De-identification: To mitigate privacy risks, techniques such as data anonymization and de-identification are employed. These methods remove or alter identifying information from datasets, making it difficult or impossible to link data back to specific individuals. This helps protect individuals’ identities while still enabling the use of data for AI research and development.
Secure Data Storage and Transmission: AI systems require secure data storage and transmission to prevent unauthorized access or breaches. Implementing robust security measures, such as encryption, access controls, and secure protocols, is essential to safeguard sensitive data throughout its lifecycle, from collection to storage and sharing.
Minimizing Data Collection and Retention: AI applications should aim to collect only the necessary data for the intended purpose and retain it for as long as required. Minimizing data collection and retention limits the potential exposure of sensitive information and reduces the risks associated with data breaches or misuse.
Ethical Use of Data: Organizations must ensure that AI systems are used ethically and responsibly, respecting individuals’ privacy rights and avoiding the exploitation or harm that may arise from the use of personal data. Clear policies, guidelines, and ethical frameworks should be in place to guide the development, deployment, and governance of AI systems.
Transparency and Accountability: Promoting transparency and accountability is crucial in maintaining trust in AI systems. Organizations should be transparent about their data practices, providing clear explanations about the data collected, how it is used, and the measures taken to protect privacy. Accountability mechanisms should be established to address any privacy breaches or misuse of data.
By addressing data privacy and security concerns, organizations can build trust with users and stakeholders, ensuring that AI systems are developed and used in a manner that respects privacy rights and upholds ethical standards.
Ai in Natural Language Processing
Artificial Intelligence (AI) has made remarkable strides in the field of Natural Language Processing (NLP), enabling computers to understand, interpret, and generate human language. AI-powered NLP systems have revolutionized various applications and industries. Here are some key aspects of AI in NLP:
Language Understanding: AI algorithms have been developed to comprehend the meaning and context of human language. Techniques like word embedding and deep learning models such as Recurrent Neural Networks (RNNs) and Transformers have significantly improved machines’ ability to analyze and understand text data. This advancement has led to applications such as sentiment analysis, topic modeling, and text classification.
Machine Translation: AI-powered NLP systems have made significant progress in machine translation. Neural machine translation models, based on deep learning techniques, have surpassed traditional rule-based and statistical methods. These models can translate text between different languages with remarkable accuracy, improving cross-lingual communication and facilitating global interactions.
Chatbots and Virtual Assistants: AI has transformed the capabilities of chatbots and virtual assistants. NLP algorithms enable these AI-powered agents to understand and respond to human queries in a conversational manner. Through natural language understanding and generation, chatbots and virtual assistants can provide personalized assistance, answer questions, and perform tasks, enhancing customer support, and user experiences.
Text Generation and Summarization: AI models have advanced text generation capabilities, including the ability to generate coherent and contextually relevant text. This has implications for applications such as automated content creation, chatbot responses, and personalized recommendations. NLP algorithms also facilitate text summarization, enabling the extraction of key information from large documents or articles.
Sentiment Analysis and Opinion Mining: AI-powered NLP techniques enable sentiment analysis and opinion mining, helping organizations analyze public sentiment towards products, services, or events. This information can be used for brand monitoring, market research, and reputation management, providing valuable insights into customer perceptions and preferences.
Named Entity Recognition and Information Extraction: AI algorithms can automatically identify, and extract named entities (such as names, organizations, locations) from text data. This facilitates information retrieval, data categorization, and knowledge extraction from large volumes of unstructured text, streamlining processes in various domains like information retrieval, content analysis, and legal document processing.
AI continues to drive advancements in Natural Language Processing, opening up new possibilities for human-computer interaction, information processing, and knowledge discovery. By leveraging AI techniques, NLP systems have become more accurate, versatile, and capable of understanding and generating human language with increasing sophistication.
FAQs
Q:What is difference between artificial intelligence and machine learning?
While artificial intelligence (AI) and machine learning (ML) are related concepts, they are not the same thing. AI refers to the broader field of computer science that focuses on creating intelligent machines capable of mimicking human intelligence and performing tasks that typically require human intelligence, such as problem-solving, decision-making, and natural language processing.
On the other hand, machine learning is a subset of AI that focuses on algorithms and statistical models that enable computers to learn and improve from experience without being explicitly programmed. ML algorithms allow machines to analyze and interpret data, identify patterns, and make predictions or decisions based on that data.
In essence, machine learning is a specific approach within the broader field of artificial intelligence. While AI encompasses a wide range of techniques and applications, machine learning specifically emphasizes the ability of machines to learn and improve from data without explicit programming. Machine learning algorithms are a key component of AI systems, enabling them to adapt and improve their performance over time.
It is important to note that AI and ML are closely interconnected and often used together. ML techniques and algorithms are frequently employed to develop and enhance AI systems, enabling them to learn and adapt from data. The combination of AI and ML enables the development of intelligent systems that can automate tasks, analyse complex data, and make informed decisions based on patterns and trends.
Q: What are some real-world applications of artificial intelligence and machine learning?
AI and ML have numerous applications across industries. In healthcare, they are used for diagnosis, treatment planning, and drug discovery. In finance, AI and ML algorithms assist in fraud detection, risk assessment, and algorithmic trading. In retail, the power recommendation systems and chatbots. In manufacturing, AI and ML optimize production processes and predict equipment failures. In transportation, they contribute to the development of autonomous vehicles and intelligent traffic management systems.
Q: How do AI and ML algorithms learn from data?
AI and ML algorithms learn from data through a process known as training. During training, the algorithms are exposed to a large amount of labeled or unlabeled data, depending on the specific learning approach.
In supervised learning, the algorithm is trained on labeled data, where each data point is associated with a corresponding label or target value. The algorithm learns to identify patterns and relationships between the input features and the target labels by adjusting its internal parameters. It iteratively compares its predictions with the actual labels and updates its parameters to minimize the prediction error. This process, known as optimization or learning, allows the algorithm to improve its performance over time.
In unsupervised learning, the algorithm is trained on unlabelled data, where there are no predefined target labels. The objective is to uncover concealed patterns, structures, or relationships within the data. The algorithm analyzes the data and identifies similarities or clusters based on the inherent patterns or statistical properties present in the data. Unsupervised learning algorithms do not receive explicit feedback or targets during training but instead learn to extract meaningful information and structure from the data.
There is also semi-supervised learning, which combines labelled and unlabelled data. This approach leverages the limited labelled data available along with a larger amount of unlabelled data to improve learning and generalization.
Once trained, the AI or ML algorithm can make predictions or decisions on new, unseen data based on the patterns and relationships it learned during training. The performance of the algorithm is typically evaluated using evaluation metrics that measure its accuracy, precision, recall, or other relevant criteria.
Q: What are some challenges in the adoption of AI and ML?
The adoption of Artificial Intelligence (AI) and Machine Learning (ML) technologies presents several challenges that organizations need to overcome. One of the primary challenges is related to the quality and availability of data. AI and ML models require high-quality, diverse, and representative data for effective training and inference. However, organizations often struggle with issues such as incomplete, inaccurate, or biased data. Obtaining sufficient and relevant data can also be a challenge, particularly in industries with limited data availability.
Another significant challenge is the scarcity of skilled professionals. AI and ML implementation demand expertise in data science, machine learning algorithms, and programming. Finding and retaining qualified professionals with the necessary knowledge and experience can be a daunting task for organizations, considering the high demand and limited supply of talent in the field.
Ethical and legal considerations pose additional challenges. The ethical implications of AI and ML, including privacy concerns, algorithmic bias, and potential job displacement, require careful attention. Organizations must navigate legal and regulatory frameworks to ensure compliance and address societal concerns related to fairness, transparency, and accountability.
Explainability and interpretability of AI and ML models present another challenge. These technologies often operate as “black boxes,” making it difficult to understand the reasoning behind their predictions or decisions. Lack of interpretability can hinder trust and acceptance, particularly in domains where human accountability is crucial. Researchers and practitioners are actively working on addressing this challenge to enhance the explainability of AI and ML outputs.
Integration and scalability pose technical challenges. Integrating AI and ML systems into existing infrastructure and workflows can be complex and time-consuming. Compatibility issues, legacy systems, and data silos need to be overcome for seamless integration. Additionally, scaling AI and ML solutions to handle large-scale data processing and real-time requirements may require significant computational resources and infrastructure upgrades.
Cost and return on investment considerations are also significant challenges. Developing and implementing AI and ML solutions involve substantial investments in technology, infrastructure, and talent. Organizations need to carefully assess the cost-benefit ratio and potential return on investment to ensure the viability and sustainability of AI and ML initiatives.
Regulatory and safety concerns are specific challenges in certain industries. Adhering to regulations, ensuring safety, and addressing potential risks and liabilities associated with AI and ML technologies require careful attention and compliance.
Despite these challenges, organizations are actively working to overcome them and leverage the potential of AI and ML. Collaboration between academia, industry, and policymakers is crucial to mitigate risks, foster responsible adoption, and realize the transformative benefits of AI and ML.
Q: What are the future trends in AI and ML?
The field of Artificial Intelligence (AI) and Machine Learning (ML) is continuously evolving, and several significant trends are shaping the future of these technologies. Here are a few significant trends to pay attention to:
One major trend is the rise of Explainable AI. As AI systems become more prevalent, there is a growing need for transparency and interpretability. Explainable AI focuses on developing models and algorithms that can provide explanations for their decisions and predictions. This trend aims to enhance trust, accountability, and understanding of AI systems, particularly in critical domains such as healthcare and finance.
Another emerging trend is Federated Learning. With increasing concerns about data privacy, federated learning has gained attention. It allows AI models to be trained on decentralized data sources without the need for data sharing. This approach ensures data privacy while still benefiting from the collective knowledge and insights derived from multiple sources.
Reinforcement Learning is also gaining traction in real-world applications. This approach enables agents to learn through interactions with an environment. Reinforcement learning is being used in areas such as robotics, autonomous vehicles, and personalized recommendation systems. As algorithms and techniques improve, reinforcement learning is expected to play a crucial role in solving complex problems and optimizing decision-making processes.
Additionally, there is a growing focus on AI ethics and responsible AI. As AI becomes more integrated into society, ethical considerations regarding bias, fairness, and accountability are gaining prominence. Organizations and researchers are actively working on developing frameworks and guidelines for ethical AI development and deployment.
The fusion of AI and other emerging technologies is also a significant trend. AI is being combined with technologies like Internet of Things (IoT), blockchain, and augmented reality to create innovative solutions. For example, AI-powered IoT devices can gather and analyze vast amounts of data, enabling smart and efficient decision-making.
Lastly, AI democratization is on the rise. Efforts are being made to make AI more accessible to a broader range of users, not just experts in the field. User-friendly AI tools, platforms, and frameworks are being developed, enabling individuals and organizations to leverage AI and ML capabilities without extensive technical expertise.
These trends collectively shape the future of AI and ML, driving advancements in transparency, privacy, ethical considerations, integration with other technologies, and accessibility. As these trends continue to evolve, we can expect AI and ML to play increasingly significant roles in transforming various industries and driving innovation.
Conclusion
Artificial intelligence and machine learning are transformative technologies that are reshaping industries and society. From healthcare to finance, retail to manufacturing, and transportation to entertainment, AI and ML are revolutionizing the way we live and work. The benefits of these technologies, such as increased efficiency, enhanced decision-making, and improved customer experiences, are driving their widespread adoption. However, challenges related to ethics, privacy, and bias need to be addressed to ensure responsible and fair use. Looking ahead, future trends in explainable AI, edge computing, and NLP present exciting opportunities for further advancements. As AI and ML continue to evolve, it is essential to embrace these technologies responsibly and harness their potential for the betterment of society.